Previously, the words “artificial intelligence” (AI) made us think of a dystopian universe ruled by robots. We have popular science fiction to thank for that. But now, when people like business executives hear the word AI, they think of opportunity.
In November 2022, the launch of ChatGPT, a conversational AI chatbot, broke the internet. Within two months of its launch, it gained the title of the fastest-growing user base, with over 100 million users.
Many businesses explored its capabilities to streamline their workflows. While some used it to generate content, others used it for process automation. And that’s just the tip of the iceberg.
Thanks to the advent of tools like DALL-E, and GitHub’s CoPilot, more companies are seeing the potential AI has to go beyond the usual “automate everything” catchphrase we’ve been hearing.
To explore its true potential in 2023, we’re going to do a deep dive into the significance of AI in business. In this article, we’ll discuss the following:
- The history of AI in business
- Popular success and failure stories
- Prominent use cases
- A mini-guide on how to adopt AI
Let’s dive in.
History of AI in business
A recent survey by PwC found that 36% of leaders already have business processes enabled by AI or are adopting AI at a large scale. However, that wasn’t always the case.
The term “artificial intelligence” was coined in 1956 by four scientists: John McCarthy, Marvin Minsky, Nathaniel Rochester, and Claude Shannon. Soon after, in 1961, Alan Turing, a famous mathematician, wrote a paper on how computers can simulate a human being's thought process and do intelligent things. This milestone marked the advent of the field of AI.
But as time passed, the scientific community published literature like the Lighthill Report and Perceptrons that was heavily critical of AI and its failure to meet previously set objectives. It led to a severe withdrawal of funding from funding bodies in the United States and the United Kingdom. After almost 20 years of significant interest in the field, it brought in the first AI winter.

Interest in AI revived again in the 1970s when expert systems were developed. These systems simulated the judgment and behavior of human experts or organizations with domain expertise. However, technological drawbacks resulted in another AI winter that lasted till the mid-1990s.
In the late 1990s, interest arose again due to significant developments in the field. IBM launched Deep Blue, which beat a human chess champion in its own game. At the same time, several research institutions were working on robots, self-driving vehicles, and more which brought in the AI spring—the longest it has ever lasted.
Now, we have AI-powered chatbots, voice cloners, meeting assistants, and more—only in two decades.
What are the most prominent use cases of AI?
With more than seven decades of progress, AI has come a long way.
Danny Pan, Head of Data Science at SetSail says, “With the explosion of AI, some tools could be perceived as gimmicky. While AI is not a fad, some tools certainly will be. It’s essential to prove a tool adds value when determining if it’s a useful asset to retain long-term.”
The only way to find out whether a tool actually adds business value is by putting it to use. Here are a few stories of successes and failures of AI in business:
- Manufacturing the perfect Cheetos: Microsoft’s Bonsai
Thanks to Microsoft's Project Bonsai, PepsiCo can always create the perfect Cheeto. This powerful AI platform uses reinforcement learning to learn from Cheetos experts.
In turn, it checks the quality of every single chip—ensuring it has the perfect size, flavor, shape, and air. It makes sure the product meets the highest quality standards with every batch they produce. This use case proved the value of AI in manufacturing.
- Serving the right series recommendations: Netflix
Netflix uses AI algorithms to analyze the type of content its customers watch. As a result, it offers personalized recommendations tailored to their interests. It's done by collecting data on customers' viewing habits and content preferences, allowing the algorithm to understand what options they would enjoy. It provides an enjoyable user experience—increasing user retention. This use case proved the value of AI in personalization and using it to reduce customer churn for software products.
- Unsafe cancer treatment recommendations: IBM Watson
IBM’s Watson for Oncology was a multimillion-dollar failure. It was only trained using a small set of hypothetical patient data instead of real-life patient information. This oversight led to disastrous consequences, with multiple examples of dangerous and incorrect treatment advice being identified. Due to its inability to live up to the intended use case, the $62 million project was eventually scrapped. This use case showed the importance of providing a large and unbiased dataset to AI models to get the right output. Small datasets don’t provide enough foundational data for the AI model to make accurate decisions.
- Corrupt Twitter chatbot: Microsoft’s Tay
The failure of Microsoft's chatbot Tay (Thinking About You) proved to be a lesson in the importance of offering correct training data. The algorithms that powered the bot were designed to learn from conversations it had with people on Twitter.
Less than 24 hours after its launch, it started tweeting offensive, hateful messages due to its interactions with internet trolls. It exposed a fundamental flaw in Tay's design: a lack of filtering systems. Microsoft has to immediately pull the plug to avoid further damage to its reputation. This use case proved that AI models should be trained using moderated data to prevent such issues. The model is only as good as the data it has been trained on.
Use cases of AI for small businesses
The real world applications of artificial intelligence are far and wide. Let’s look at how small businesses can leverage AI:
Automate lead scoring and management
Small businesses can use conversational AI chatbots to capture lead information and add it to customer relationship management software (CRM). This technology can save time, increase efficiency, and improve customer satisfaction.
You can program it to ask questions like name, email address, phone number, or other desired data points. You can also add lead qualification questions like the type of business, budget, and more. These answers can then be automatically transferred into the company's CRM—eliminating the need for manual data entry.
In addition, you can integrate AI tools for business like ChatGPT with your lead management system. You can train the ML model based on internal data like average time spent on page, number of clicks, social media interactions, device type, and demographics. Based on that, it can score leads and determine which of them is likely to convert better.
Capture meeting notes automatically
Many people spend too much time in meetings. Yet, they’re constantly context switching between taking notes and trying to listen to other participants. To avoid this, you can use AI-powered solutions like Supernormal to make the most of your meetings.
For instance, it can record the entire meeting and capture short highlights which reflect the important minutes in your call. Plus, you can transcribe the call and create in-depth notes and send it to relevant participants.
Streamline content workflows
Small businesses often have limited resources and staff. This makes creating content at scale for digital marketing campaigns difficult. Fortunately, AI tools can quickly generate high-quality content that is both engaging and persuasive.
From writing to editing to optimization, there are several AI tools for businesses available for each part of your content workflow. You can generate blog posts using AI writing tools that have templates or workflows to help you get started.
After you’ve generated the copy, you can run it through an AI editor that analyzes the copy for grammatical errors, adherence to style guidelines, and more. The goal is to reduce the amount of time it takes to produce a content asset—while maintaining quality and keeping costs down.
Draft emails and subject lines for various purposes
Using AI tools like ChatGPT, sales and marketing teams can write compelling cold emails and optimized subject lines in minutes. All you have to do is provide a provide a prompt that has the following details:
- Intended recipient
- Purpose of the email
- Personalization info
- Pain points
- Call to action
Based on this input, it can generate a cold email and subject line that you tweak further based on your preferences. This lets sales and marketing teams focus on research and relationship building, instead of writing the email. It’s also useful for creating canned responses for administrative and support tasks.
Screen potential candidates
You can automate the hiring process, from resume screening and applicant tracking to interview scheduling. AI tools can automate manual human resources tasks such as resume sorting. Plus, it can analyze the responses and recommend who to hire if trained on unbiased data.
In the interview stage, you can automate tasks like sending a scheduling email, a follow-up email, and an analysis of call recordings. This approach saves hiring managers time, hastens the hiring process, and allows them to focus on high-level work.
Use cases of AI for corporations
In their recent book, Henry A. Kissinger (Chairman of Kissinger Associates), Eric Schmidt (ex-Google CEO), and Daniel Huttenlocher (dean of the MIT Schwarzman College of Computing) say, “Because of the expertise and computing resources needed to train machine-learning models, creating an AI requires the resources of large companies or nation-states.”
This is true as larger corporations have access to umpteen resources, making it easier to leverage the true potential of AI. It's also why their use cases are more extensive in scope and customized to their needs. Here are a few examples of the same:
Ecommerce personalization
Large corporations already use AI to personalize customer recommendations and increase their bottom line. Companies like Alibaba use in-house personalization algorithms to analyze customer data and generate tailored recommendations. This level of personalization keeps customers coming back, which is evident from the fact it made $129.98 billion in revenue in 2022.
Insurance claims processing
When it comes to more serious use cases of AI, insurance claims processing is a significant one. Previously, customers had to wait days or even weeks to process their insurance claims. In some cases, they don't get back half of what they expected, leading to distrust in the company.
Companies like Bdeo solve this issue using AI. Its product can conduct a thorough assessment through images and video files to collect evidence, assess damages, and provide accurate repair cost estimates in minutes. It can also be used for fraud detection. It results in a win-win situation for the insurer and customer—leading to better processes.
AI-based ecosystems for multiple use cases
Companies such as Airbus have created their own AI-based ecosystem to power their operations. Some of the use cases include:
- Optimization of flight routes of their aircraft
- Reduce fuel usage
- Conduct predictive maintenance on their planes
- Use autonomous flight systems
- Robotic assistants for astronauts
- Optimizing catering services to drive sustainability
It's possible through real-time data streams from the aircraft while it's in the air. These models analyze their systems and hasten the decision making process. For example, predictive maintenance helps identify potential issues before they happen, reducing operational costs.
How to incrementally adopt AI throughout your business
Now that you're aware of the multiple use cases depending on the size of the company, let's look at how you can start adopting AI in your organization:
Step 1: Determine what problems you need to solve
To start with AI, you must first understand what your business needs. Too often, companies tend to think of it backward. They want AI to solve problems—even if they’re not sure what problem it needs to solve.
In his book Smart Until It’s Dumb, Emmanuel Maggiori, a freelance software engineer, says, "In an ideal world, one has a problem first and finds the best solution for it afterward. However, in the world of AI, very often, people first bring the solution to the table—AI—without even knowing what they'll do with it."
Think about what problems you need to solve. Do you want to automate your workflows? Or are you looking for higher-level output with existing data? Based on that, narrow down a product that will add real value to your business.
Here’s a framework by PwC that shows how forward-thinking AI business leaders are choosing a product:
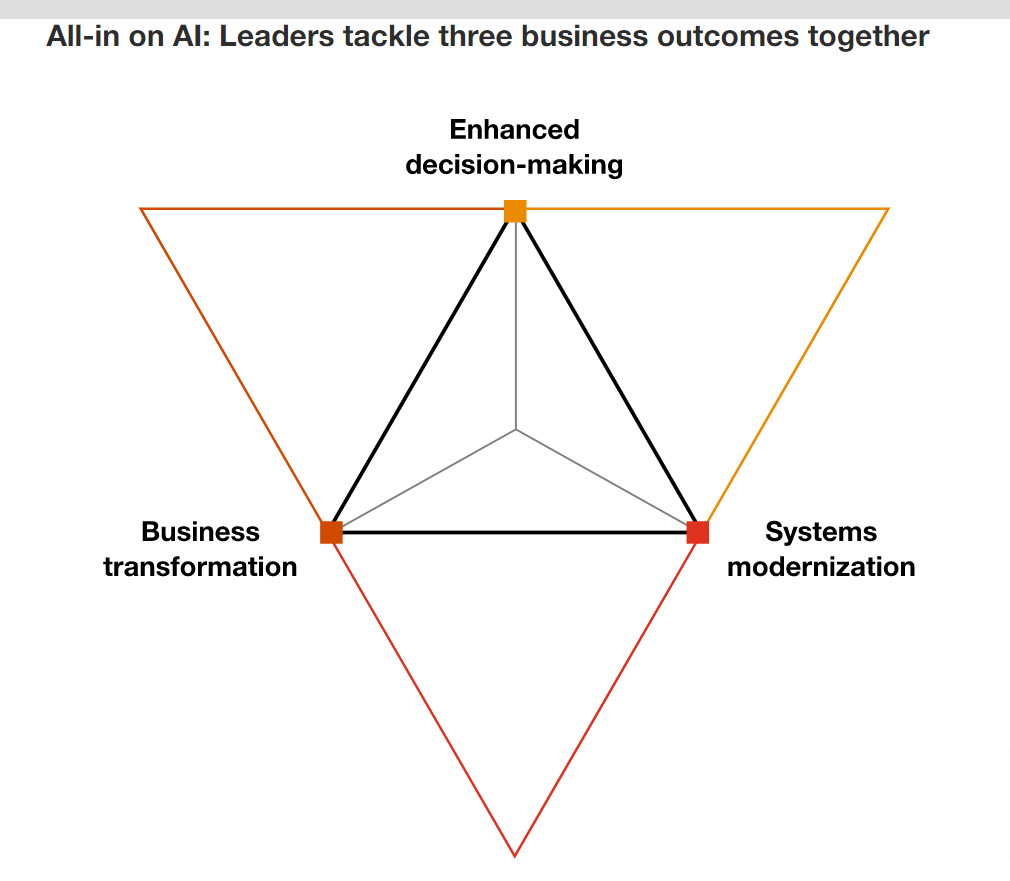
Step 2: Speak to AI experts before implementing it
Once you have an idea of your business needs, consult an AI expert. They identify potential pitfalls and create strategies to avoid them.
Athina Zisi, Chief Operating Officer at Energy Casino says, “We sought feedback and advice from experts and other organizations who had implemented similar tools. We ensured that the implementation of AI-powered tools didn't negatively impact our workforce and that it augmented and enhanced our team's capabilities.”
Plus, expert providers can recommend AI systems that would best suit the company's needs, keeping ethical implications at a minimum and providing a positive customer experience.
Step 3: Conduct a cost-benefit analysis
Once you know what you're looking for, conduct a cost-benefit analysis of your options. It should help you understand if the tool is worth the investment.
Pan says, “AI tools should go through the same evaluation process as any other tool. AI tools, like all tools, should add quantifiable value to the business.”
Think about the long-term impacts of adopting AI solutions, too. You need to consider immediate financial returns and any future savings or opportunities investing in AI offers.
Milosz Krasinski, Managing Director at Chilli Fruit Web Consulting, offers a handy tip. He says, "I suggest small business owners check out as many free trials as they can. Testing their abilities might be a good place to see what is useful and important for YOUR company."
Step 4: Implement AI-powered tools and monitor progress
When companies adopt AI, it's crucial that they not only implement the tools but also monitor its progress.
Giorgio Daher, a member of the advisory board at Headline Bulletin, says there are several challenges to adopting AI. Here are some examples of challenges:
- Not understanding the distinction between narrow and broad AI
- Not having clean and well-managed data to feed into the AI (No matter how intelligent AI is, if you are feeding it bad data, it will give you inadequate insights)
- Ethical concerns (E.g., if you use AI for risk and compliance, who is accountable if there is a breach or an error?)
To avoid this, regularly evaluate performance, adjust models, and create feedback loops to improve accuracy. As you keep adjusting, you’ll be able to ensure that the tool works well in your business context.
Step 5: Analyze, iterate, and scale its usage
It’s crucial to take an incremental approach instead of expecting widespread usage. When you do, you can prove the value of AI by testing it on a small sample of their big datasets before expanding significantly.
It allows you to track progress and make iterative improvements to maximize usage. The key is to start small, focus on specific tasks or problems, and continually monitor performance while looking for ways to optimize the use of AI further.
The future of AI in business
It's clear that despite the AI winters we've seen in the past, AI is rapidly becoming the norm for businesses of all sizes. From its early beginnings in simulations to today's powerful algorithms, it has become a crucial part of business operations. Its use cases will only increase as more data becomes available and better tools are developed.
Salva Jovells, Marketing Manager at Hockerty, believes that the future of AI would include open APIs so they can be integrated and customized, education on how to use them, and regulation on its limits.
On the other hand, Daher believes that AI will continue to go through "winters" and "springs." He says, “The notion that it will replace humans is far fetched. I think AI will augment human capabilities allowing humans to dedicate their much more nuanced intelligence to higher value endeavors.”
This is the longest period of sustained interest we've seen in AI technology. While there's a lot of potential, we still need to evaluate the risks of AI in its entirety. However, to make the business case for AI, it’s best that you understand its capabilities first and then leverage it for your use case to gain a competitive advantage.